Our Team
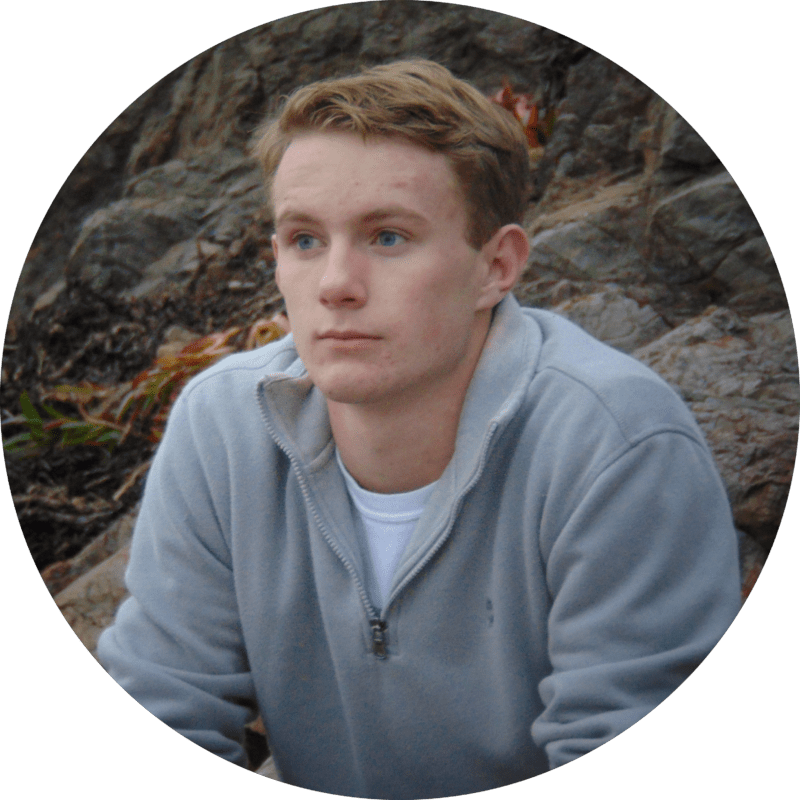
Roger Chevalier
Technical Specialist
Roger Chevalier is an Industrial Engineering Graduate from Cal Poly, San Luis Obispo. With internship experience related to facilities engineering in the wine industry, he will now be working for Nefab Packaging, starting as a Operations Global Trainee.
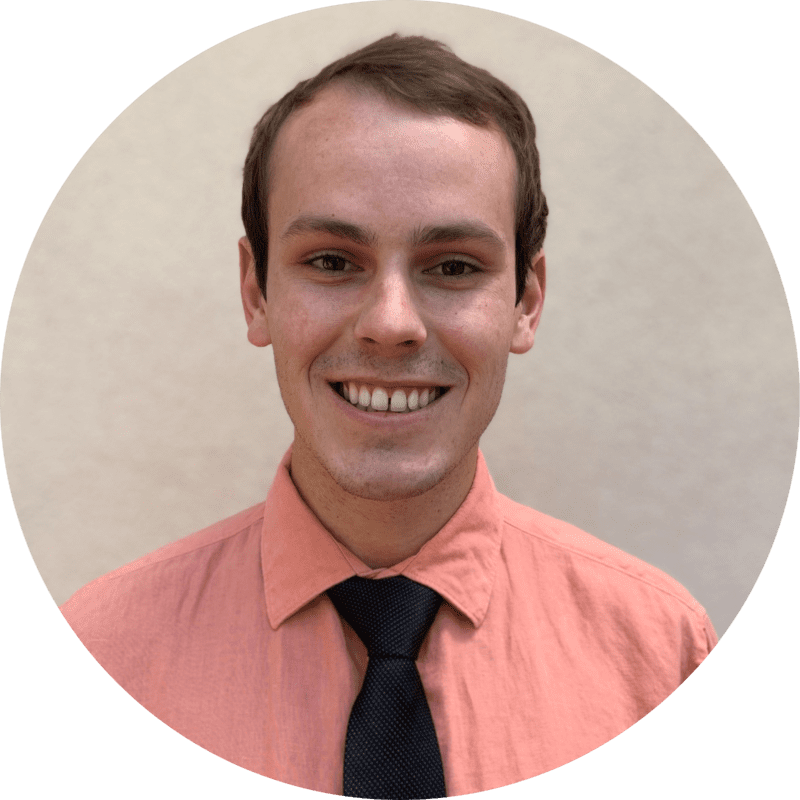
Eric Kuhlmann
Financial Analyst
Eric Kuhlmann is a 4th year student at California Polytechnic State University, San Luis Obispo. He will be graduating in Spring 2021, with a Bachelor of Science in Industrial Engineering. He is currently a quality engineering intern at Keysight Technologies and will be working as a technology consultant at RSM after graduating.
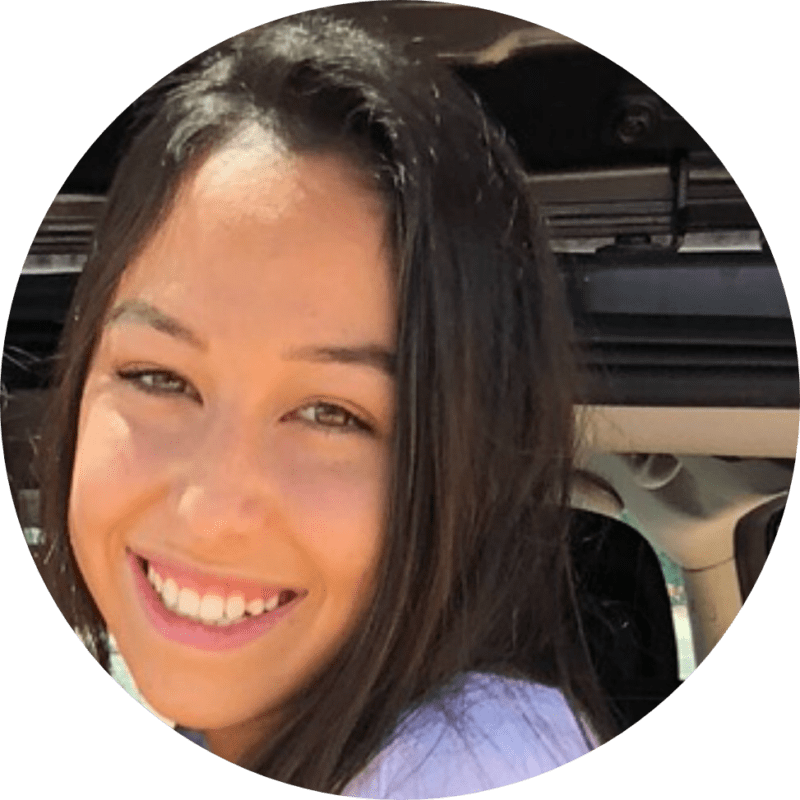
Aspen Reed
Project Manager
Aspen Reed is an undergraduate student at California Polytechnic State University, San Luis Obispo. She will be graduating in June 2021, with a Bachelor of Science degree in Industrial Engineering.
Acknowledgements
We would like to thank our project sponsor, Jonathan Polly, for bringing this project together and helping us gain contact with many corproations throughout the project. Additionally, Lucas Funes and Francisco Vonscheidt from Webee for providing the software and the IT support for this project, and Aaron Lee from Yokogawa for providing the sensors. Finally, Tali Freed for mentoring us throughout the entirety of the project
Abstract
This project was sponsored by Chevron, which is a multi-billion-dollar corporation in the oil and gas industry. The purpose of the project was to address inconsequential data collection on the Kern River oil field. The current state on the Kern River field lacked efficiency in data collection and had costs associated with the inefficient data collection, the associated costs in labor, and lack of analyzing data. Through review of the digitization in the oil and gas sector, industrial internet of things hardware and software, and use cases of low-power, wide-area network protocols (LoRaWAN) three possible solutions were developed. First, developing a SOP for pipeline inspections, Industrial Internet of Things (IIoT) operator toolkit, and a digital twin. We weighted the upfront investment, usability, and annual return in an analytical hierarchy decision matrix. Additionally, financial analysis proved the IIoT operator toolkit to be a viable solution for Chevron. After researching various software and sensor solutions, we chose Webee as our user-interface software platform and Yokogawa as our industrial grade sensor supplier. Verification and validation of the IIoT operator toolkit was completed with both simulation and accompanying analysis, as well as integration of chosen software and hardware on a conveyer belt system on the Cal Poly San Luis Obispo campus. An implementation plan with estimated timeline, costs, and resource allocation was presented. The implementation plan covers four distinct stages spanning the duration of 3 years. The final conclusions suggested the implementation of our IIoT operator toolkit, in order to allow Chevron to benefit from the tangible monetary and operational benefits that accompany the solution. For future improvements it is also suggested to work with the LoRa Alliance to bring together the major industrial bodies such as Inductive automation and sensor manufactures to develop a standard parser and data communication method to allow for easier onboarding within the industrial space.
Our Project Video
This video was the final report presentation given to the Industrial Engineering Advisory Board and our Project Sponsors.
Our Project's Digital Poster
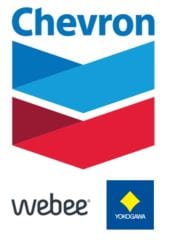
This project is sponsored by Chevron, Webee, and Yokogawa
Problem Statement
- The Kern River oil field has reached stable oil output and is focused on low-cost performance improvement strategies in order to grow operations.
- An estimated $54 million in costs associated with inefficient data collection and lack of accompanying analytics.
- $10.8 million of this is due to wasteful operator tasks and unexpected machine failures due to lack of data or poor data communication.
Current State
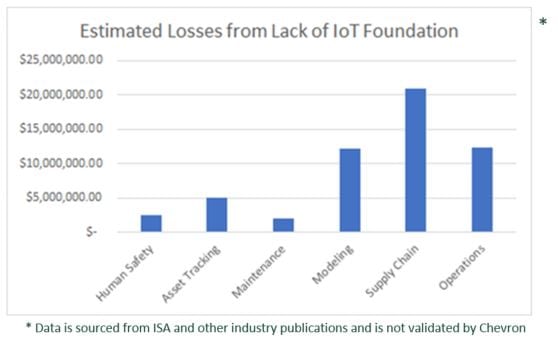
- Manual data collection
- Minimal existing usage of sensors and data analytics
- Older infrastructure relies on slow protocols
- Total losses of over $10.8 million per oil field
Project Objectives
Operations
- Duties and Tasks should be improved
- Risk of Human Error Minimized
- Operator Usability Maximized
- Meets Technical and Legal requirements of Oil Industry
Costs
- Low Upfront Investment
- Maximize Annual Return
- Decrease Operational and Labor Costs
Beyond
- Scalability
- Sustainability
Literature Review
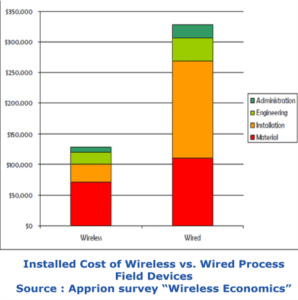
Our research covered the 4th Industrial Revolution Challenges, Reducing Operational Costs, Wide Area Networks, Industrial Sensors, Industrial Internet of Things Software Solutions, and the Digitization of Oil and Gas.
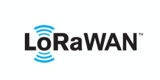
Network- LoRaWAN was chosen because of its emphasis on low power usage and wide area capabilities.
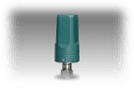
Sensor- Yokogawa Sushi Sensor was chosen due to its long battery life and its Zone-1 qualifications
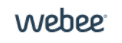
Software- Webee was chosen due to its scalability, agnostic nature, and focus on low coding solutions
Potential Solutions
Standard Operating Procedure and Route Optimization
This solution is focused around creating SOP that are more heavily focused on efficiency and uniformity in data collection. It also carries with it the implementation of a route optimization algorithm that would reduce travel time. This solution has low sophistication, but also provides low benefit and would likely face operator pushback.
IIoT Operator ToolKit
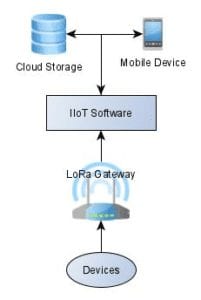
This solution implements an inventory of sensors working through a LoRaWAN gateway and a software solution to allow for rapidly deployable, operator friendly digital data collection solutions. It uses low cost equipment, long battery life, and an expandable network to address the problem.
Digital Twin
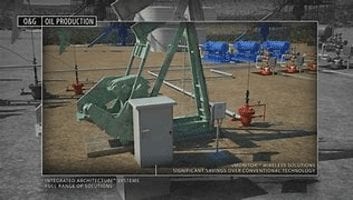
This Solution deploys a full scale sensor system and the creation of a digital twin. This solution would address all possible areas of data collection and utilization on the brown field, however it is a very costly process that could take years. This would also almost entirely remove operators.
Solution Selection
Based on the objectives described, an Analytical Hierarchal Process was carried out to decide on the best possible solution. Based on the variations in reductions in labor, and improvements in data collection of the three solutions, Operator Usability, Investment, and Cost Benefit Ratio were all compared.
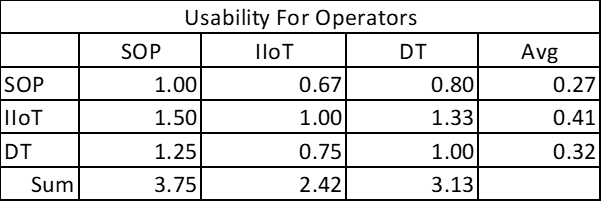
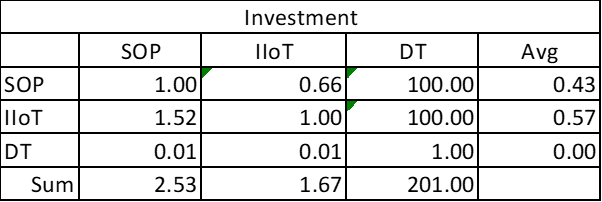
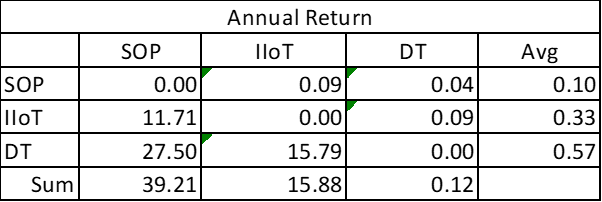
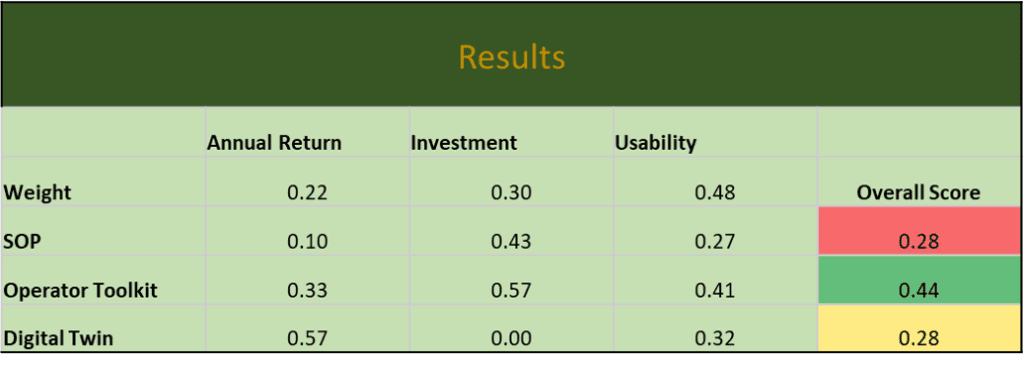
Based on or AHP as well as a thorough review of the non monetary implications of each solution, the IIoT Operator Toolkit was selected.
Impact
Qualitative:
- Sustainability- reduce power consumption and emissions
- Diversity- Allow for a operators to complete more work reducing need for heavily educated workers
- Ethical- Improve safety and decision making
Quantitative
- NPV- $16 Million
- Payback Period- 325 Days
- Cost/Benefit Ratio- 9.37
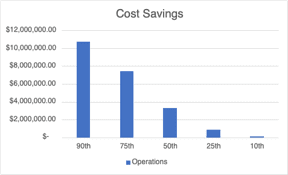
Verification
Process

In order to test this solution a flow for how deployment of new digital solutions could be deployed was created. Then the process was followed in addressing a steam pipe line clearing process on the brown field.
Simulation
The Steam Pipe Line clearing Process consists of an operators powering-down a well, turning on steam, and then guessing when to return to the pipeline to identify it as being cleared. The guessing in this process can lead to hours of wasted steam sometimes which comes with heavy fines and expensive in the industry. To address this, the simulation shows the improvement when an operator is alerted when the process is done.
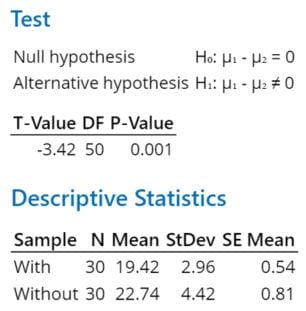
Based on the simulation created, 30 trials were run with and without the solution deployed, and as can be seen, the solution was able to reduce operation time and in turn reduce steam costs.
Steam Pipeline Cleaning
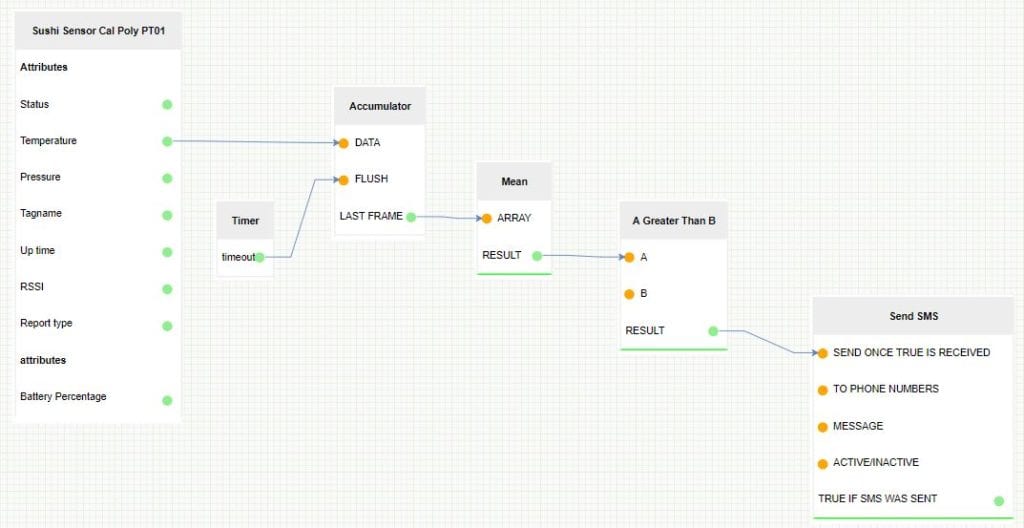
In order to test this process, first the network and sensors were configured and a simple logic flow was created to send a text notification whenever the temperature reaches above a given threshold. This flow took around 10 minutes to produce given an hour of training.
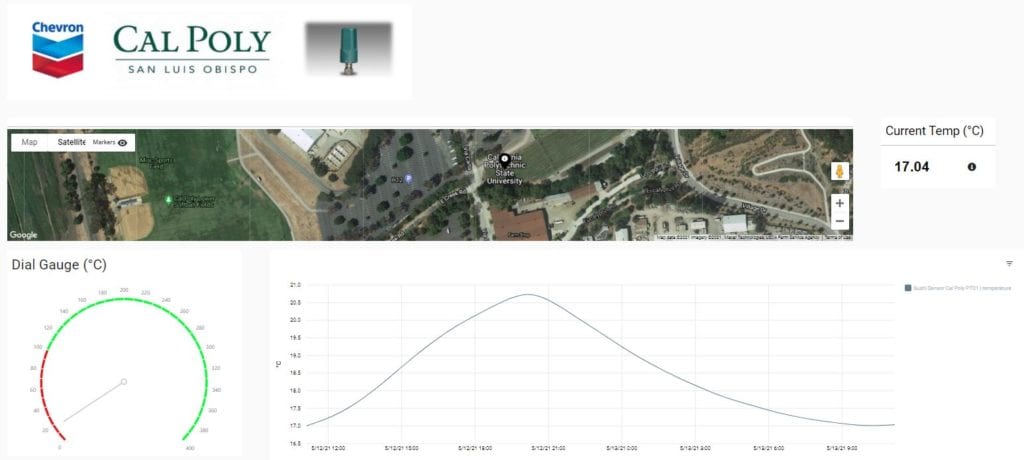
To better visual the solution, a dashboard was created, which although more design heavy, allowed for the implementation of a location logging system to track where sensors are placed as well as display historical data to understand how long the steam may have been running clear.
Implementation
Phase 1.1
Buy sensors and build inventory system
Phase 1.2
Train operators in software (2-4 hours)
Train Engineers in software (3-6 hours)
Phase 1.3
Deploy Sensors on high maintenance risk equipment such as heat exchangers, pumps, and steam traps.
Begin using Pipeline cleaning flow
Phase 2
Deploy Predictive Maintenance AI and begin to integrate data collection into current database software’s.
Conclusions
Industry 4.0 and the role of the Industrial Internet of Things has been growing rapidly. With battery life improving, sensors and software becoming easier to use and longer lasting, these solution are becoming cheaper than they have ever been before. Because of this rapid growth, there is a need now more than ever before for a standardization within the IIoT space. The use of unique parsers, unique device configuration, and other challenges that come from having so many individual players in such a new space, has resulted in increased engineering and deployment costs. If the industry can find a more standardized approach to deployment and data configuration, not only will IIoT become cheaper, it will no longer require the expensive computer engineering overhead that is often associated with these types of projects.