Our Team
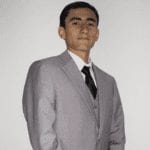
Steven Alvarado
Research Lead
Steven is a senior manufacturing engineering student graduating Winter 2022. As a central coast native, he has developed a strong liking for the outdoors and enjoys exploring new hiking trails and natural landscapes. Steven plans to intern as a manufacturing engineer this coming summer and plans on returning to the central coast to work for a local manufacturing company.
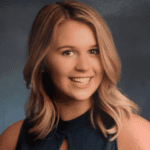
Lilly Brown
Dashboard Design Lead
Lilly Brown is a Chicago-native graduating from Cal Poly this summer with a Bachelor’s in Industrial Engineering. She will go on to work for NetSuite in Austin this fall.
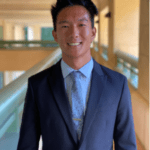
Team Member
Project Manager
Thomas Kim is an Industrial Engineering student and will be graduating from Cal Poly Winter of 2022. He is from San Diego and really enjoys the ocean and the outdoors. He worked as the Project Manager, coordinating tasks and meetings with their sponsor.
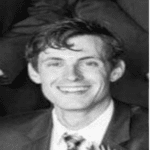
Ryan Reynolds
Impact Analysis Lead
Ryan Reynolds is a first year Industrial Engineering transfer student graduating in Winter of 2022. Although he completed most of his studies at Virginia Tech, Ryan has found a new home at Cal Poly as a mustang. With a wide range of interests in industrial engineering, Ryan focused on making sure the team was moving forward and staying well within the bounds of milestone deadlines, as well as providing occasional comedic relief.
Acknowledgements
We would like to thank our senior project sponsor, Morgan Boyd, and our senior project advisor, Jill Speece, for their continued support and guidance throughout the senior project sequence. We would also like to thank supporting personnel of the SLO Veteran Services Office for their support. We also want to thank the SLO District Attorney’s Analysts for providing supplemental resources and support to help our team navigate the county databases.
Problem Statement
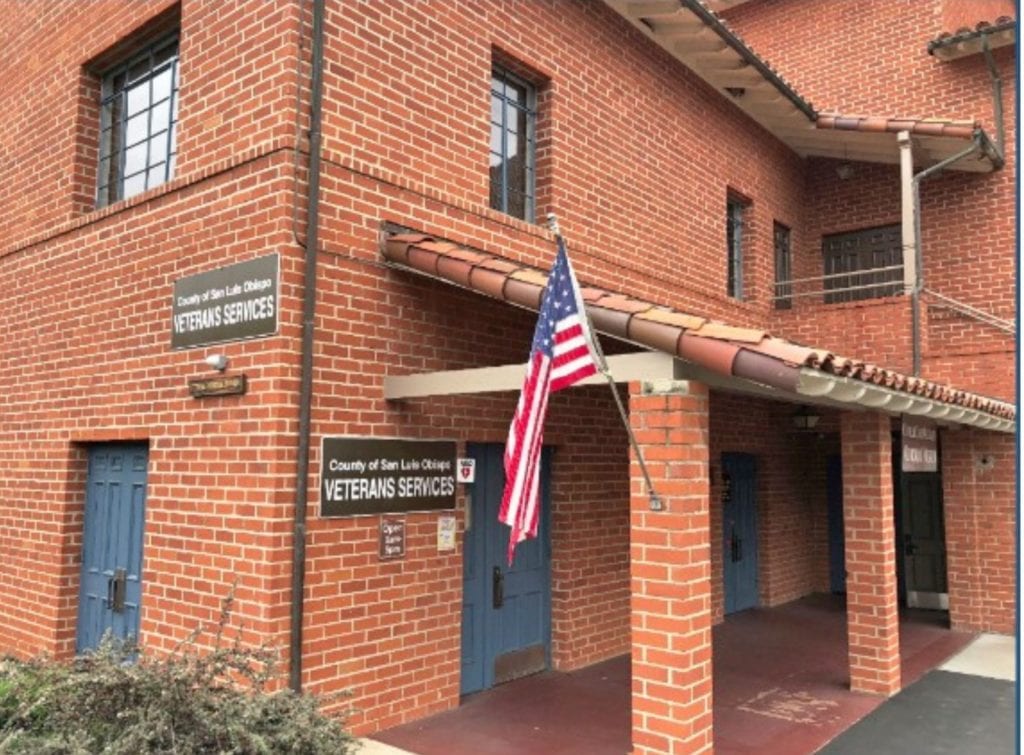
SLO County would like to improve the lives of veterans in the criminal justice system by giving them the option of treatment court, opposed to traditional court. However, the Veteran Service Office is facing a lack of efficiency with data storage and input.
Background
-
Veteran Treatment Courts (VTC) are an alternative sentencing program for criminal defendants that have served in the active military, naval, or air force services. These treatment courts help veterans with their conditions through rehabilitation and counseling. Veteran Treatment Courts are modeled after drug & alcohol and mental health courts. Treatment courts have proven to be effective year after year, helping their clients trace their problems to the root cause and giving them the appropriate treatment.
Literature Review
The aim of the literature review is to gain context of the elements that cause inefficiency within the veteran treatment court (VTC) system, as well as spark ideas for potential solutions. Currently, there are 461 treatment courts across the nations that focus solely on serving veterans with a true goal of rehabilitation and compassion instead of blind punishment. Veteran treatment courts have improved homelessness, employment, crime rates, and relationships in many communities. As shown in prior research, the national graduation rates of treatment courts are right around 75%. Across the country, each VTC operates slightly differently, however most of the California VTCs follow an 18 month plan, split into four phases.
Project Objective
Goals
The goal of this project is to improve effectiveness and efficiency within the SLO County VTC. In order to accomplish this, the team will analyze raw data to: determine the proportion of successful program participants, identify missed client opportunities, isolate and determine circumstances leading to client expulsion, and track recidivism rates on 1,3, and 5 year ranges after successful program completion. As well as making the data easier to understand, the team also will create a predictive model to help the VSO with future decision making. With a large data set, it is not expected to contain all pertinent information needed to answer every possible question. In conjunction with available KPIs, the analysis is expected to produce a list of program KPIs that would ideally be present. This will give stakeholders a great starting point to improve data collection moving forward.
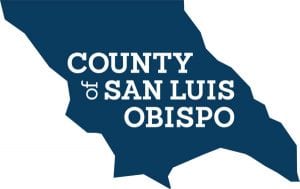
This project is sponsored by the Veteran
Services Office in the County of San Luis
Obispo, California.
Current State
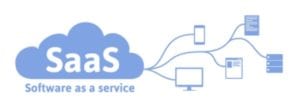
The District Attorney’s office houses and access their data through SASS
After a few weeks of rigorous data extraction, a clear picture of the SLO Veteran Treatment Court developed. The data is collected by the court, which generates a lot of data not relevant to VTC with a few gaps. Luckily, the gaps were filled by files sent by the Probation Department. Developing a link between the two databases finally revealed the graduation rates. The data provided shows that of the 170 clients who were referred to treatment court, only 42 entered. This highlights a lag in the referral process and throughput. On a positive note, the data did show that 33 successful graduates, placing the graduation rate at 79%. As shown in prior research, the national graduation rates of treatment courts are right around 75%. A success rate that is above the national average proves the importance of this program, and the positive impact it has on the community. In order to bypass the need for additional Probation Department data the team is currently pursuing the addition of a few additional event codes to the system that will allow for direct and clear indication of program participant success.
Solution Design
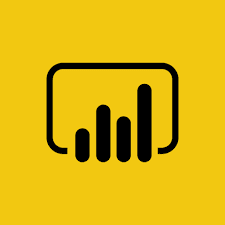
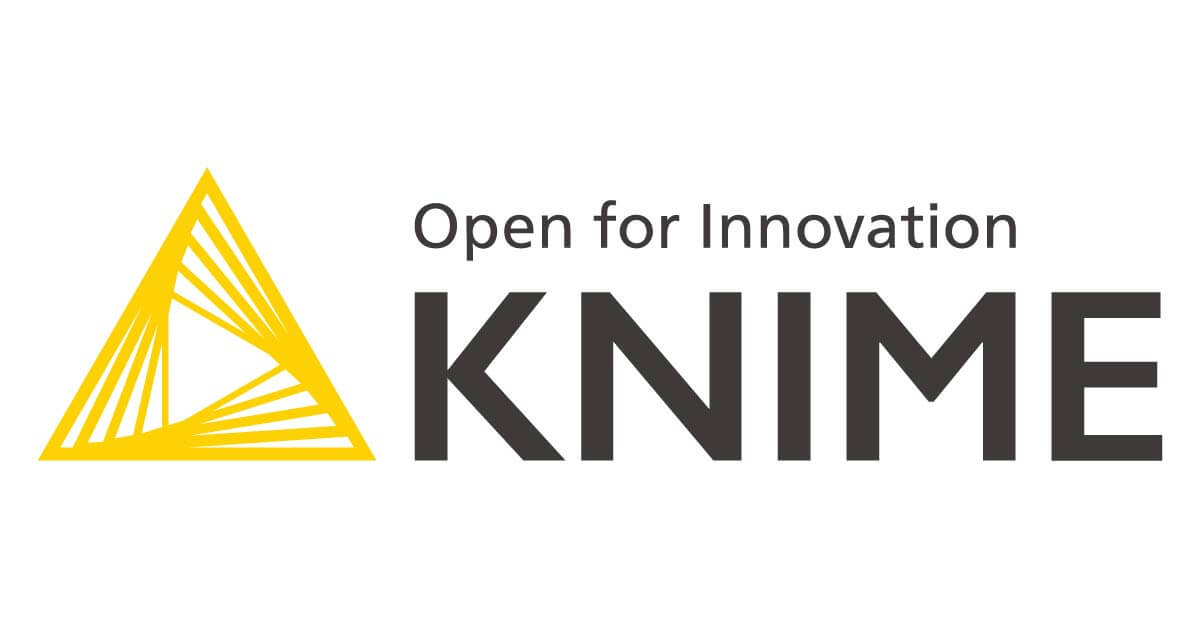
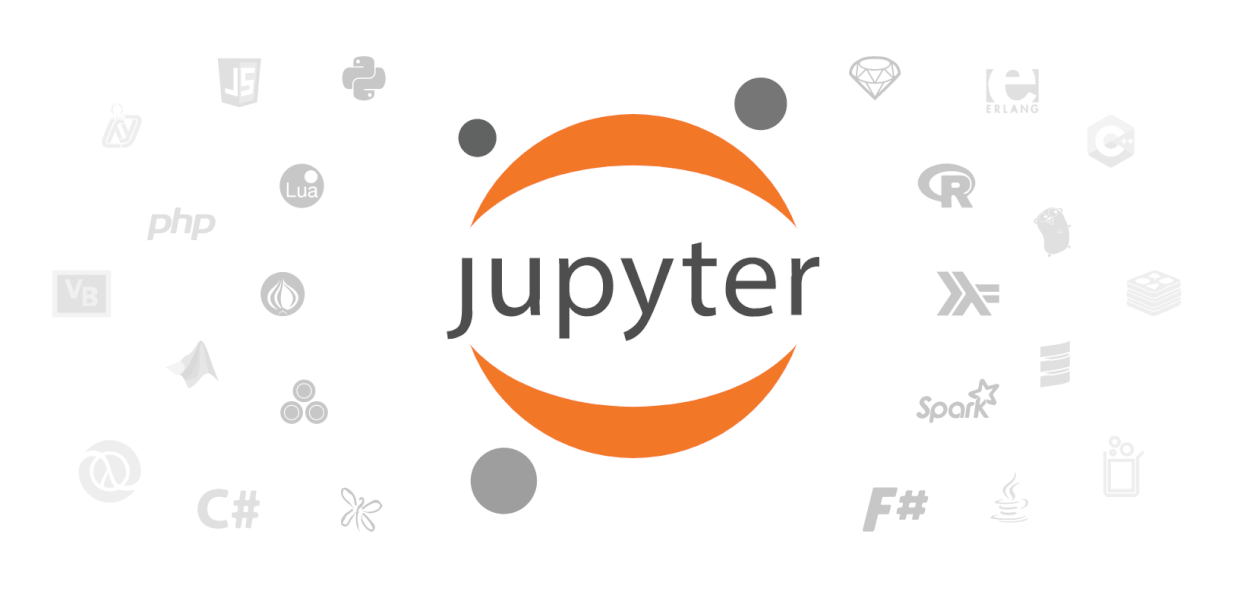
The solution was designed using PowerBI, KNIME, and and Jupyter. KNIME is a free and open-source data analytics and integration software.
Process of Design
The implementation of Microsoft PowerBI in conjunction with the SQL Server Management Studio has shown promising signs of allowing the SLO County VSO to quickly make decisions based on current data available. Live connections to the SQL database server result in continuous updates to the PowerBI graphs, charts, and other summarizing features. Early stages of the PowerBI application show great promise as SLO County Treatment Court program participants can easily be filtered on a yearly basis, and the number of program graduates can quickly be compared to the number of enrolled clients. The dashboard provides end users with a quick and intuitive way to look at the raw data as a whole and to be able to make decisions based upon patterns and other realizations made possible by PowerBI’s data analytics.
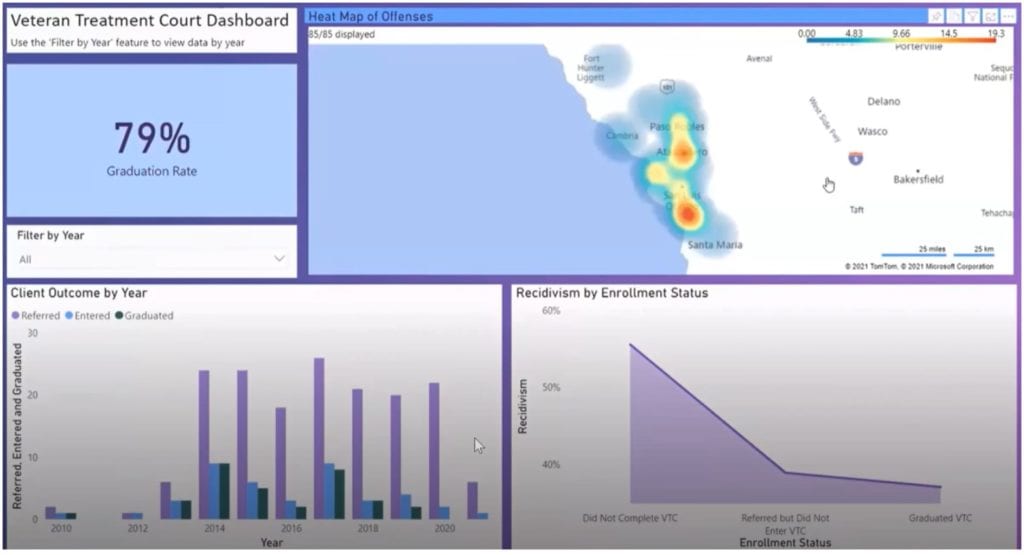
Root Cause
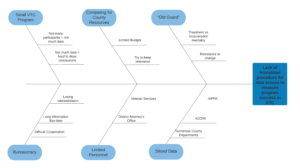
The team determined that the two main contributing factors of inefficient data access are attributed to fragmented data within the county in addition to federal laws protecting personal client data. These two factors limit data access and as a result, affect the ability of SLO County personnel to extrapolate data to be able to analyze the data and interpret findings.
Impact Analysis
Graduation Rates – The dashboard summarizes graduation rates and allows users to filter by single or multiple years.
Heat Map – The heat map feature assists the VSO in identifying high-crime areas so they can find and support new clients in the system.
Recidivism – The dashboard also shows recidivism rates of those who did and did not enter the program. This is a key metric in determining program success.
Self-Sustaining- The dashboard has a live connection to the DA’s database, meaning that there is little to no upkeep
Current State
The project scope has been expanded to include dashboard refinement, and predictive models for those entering the SLO County criminal justice system. The dashboard has been expanded to include additional key metrics and will be formulated to allow for the transition of dashboard maintenance to the Veteran Services Office personnel.
The predictive model has been built using the KNIME data analytics software in conjunction with the Python programming language. KNME has node based flow which allows for easy interpretation similar to AnyLogic. The SQL Server Management Studio was connected to KNIME creating a live connection feed. With this live feed, it now has access to all the tables in the SQL servers. Using individual KNIME nodes, the data is preprocessed and all missing and all missing values are replaced or removed from the table. After all missing values are dealt with it is then moved to Python Script Node where the table is turned into data frame. Once the Python script is executed the data is normalized and partitioned into training and testing data. Once partitioned it is ran though a decision tree node where it tells the results.
Validation
To validate the model, a receiver operating characteristic curve was conducted to make sure the model was not overfitting the data. After conducting the test, the AUC value was .73 which is considered acceptable.
There are opportunities for improved program efficiency if adjacent county organizations are able to seamlessly share information and data between organizations. This project begins to illustrate the need for increased collaboration between SLO County departments in a collective effort to support the needs of the community as a collective. The predictive model results should be taken lightly as there are many other factors that go into someone reoffending or not such as if they have a support group like family, religious or counseling group.
Conclusion
Graduation Rates – The dashboard summarizes graduation rates and allows users to filter by single or multiple years.
Heat Map – The heat map feature assists the VSO in identifying high-crime areas so they can find and support new clients in the system.
Recidivism – The dashboard also shows recidivism rates of those who did and did not enter the program. This is a key metric in determining program success.
Predictive Model – The predictive model will help the VSO make important decisions when it comes to recommending subjects to do treatment court. Something to take in mind about the predictive model is that there are a lot of factors that go into the likelihood that someone will reoffend other than someone’s past history.